Multimodal data pipelines to drive all of your
AI Workflows
Securely leverage data from any source to deliver RAG, reinforcement learning or fine tuning workflows that unlock the power of AI for your organization.
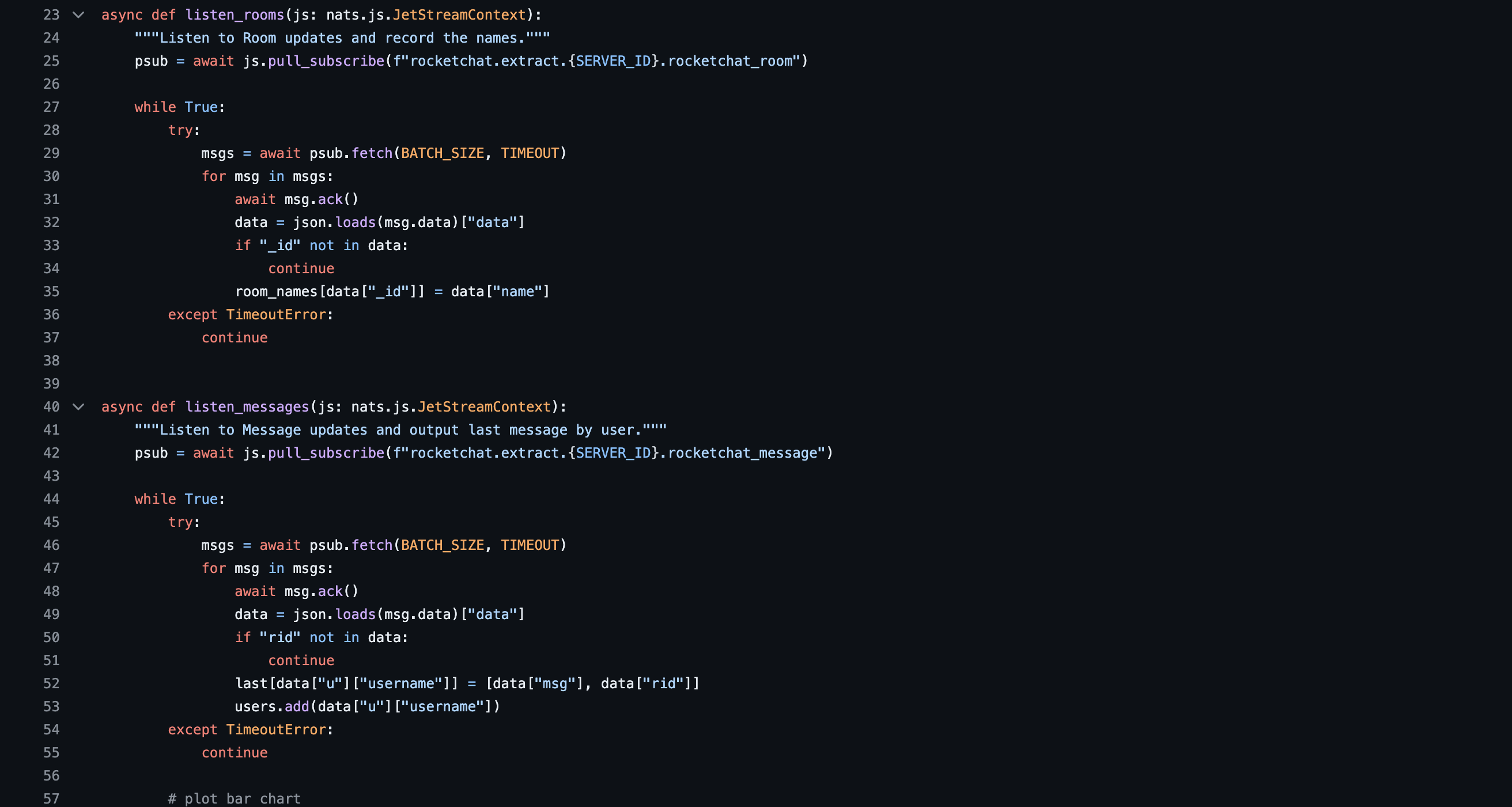
Consume Data from any source with streaming data processing or scheduled batches
Feture List Headline
Real-Time Collaboration
Enable developers to collaborate seamlessly on code projects, with features like version control, code sharing, and synchronized development environments.
Code Refactoring
Automatically optimize and restructure existing code for better performance, maintainability, and adherence to coding standards
Documentation Assistance
Generate in-line comments, documentation, and explanations to improve code readability and make it easier for developers to understand and maintain.
Code Debugging
Detect and highlight potential issues, errors, or inefficiencies in your code, making it easier to identify and resolve problems.
Intelligent Code
Enhance your coding speed and accuracy with context-aware code suggestions and auto-completion recommendations.
Auto Code Generation
Automatically generate code snippets, functions, or even entire modules based on your requirements, reducing coding time significantly.
Value Statement 1
Lorem ipsum dolor sit amet, consectetur adipiscing elit. Suspendisse varius enim in eros elementum tristique. Duis cursus, mi quis viverra ornare, eros dolor interdum nulla, ut commodo diam libero vitae erat.
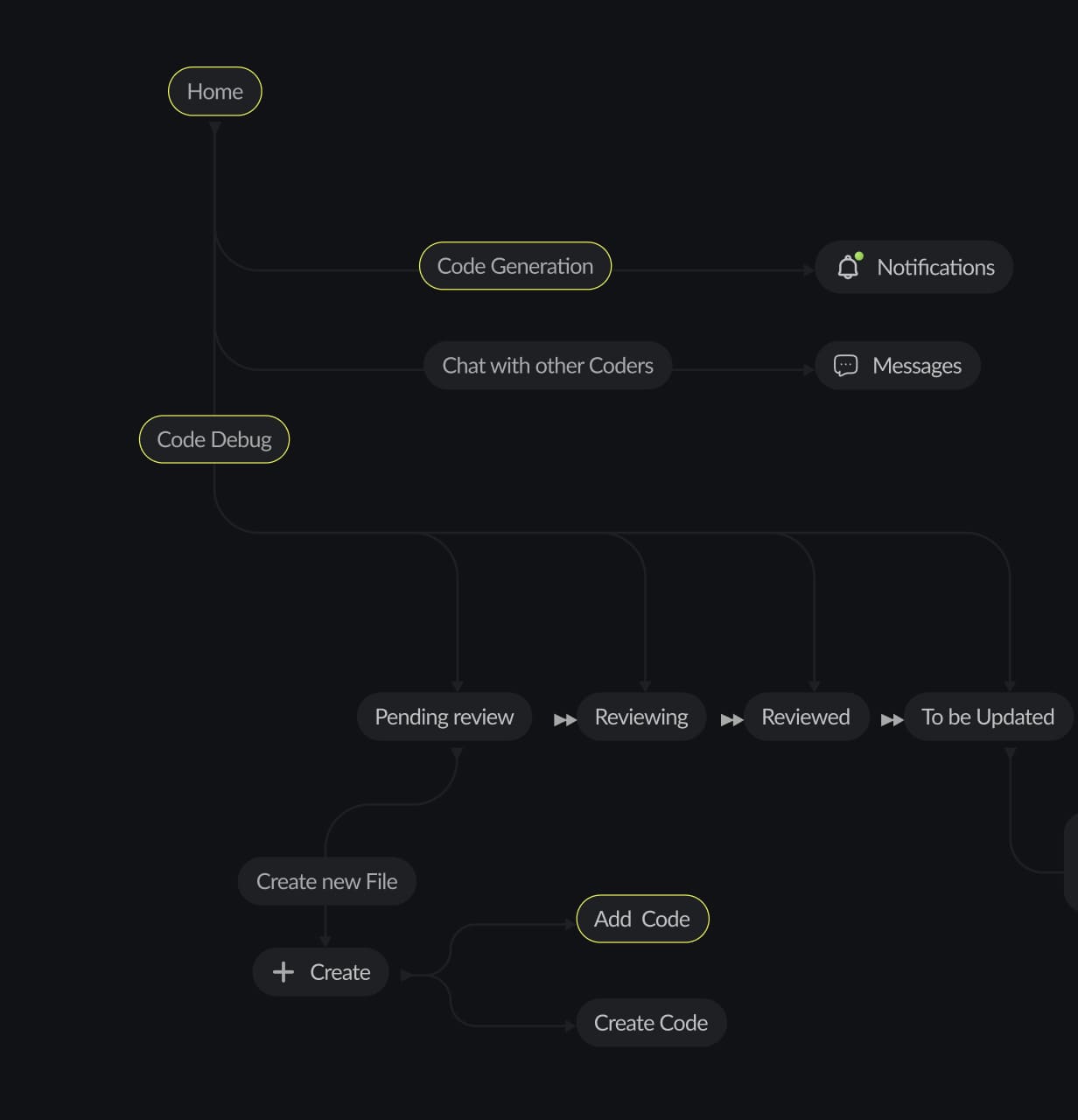
FAQs
Learn more about why our customer's work with fourthlock data pipelines
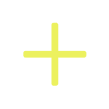
Retrieval-augmented generation (RAG) is a technique that provides generative AI large language models (LLMs) with additional data so they can deliver more relevant and useful results. As business users deepen their use of generative AI for solving real problems related to their organizatons, they often find themselves frustrated by incorrect or irrelevant answers returned by an LLM. RAG can minimize these frustrations by improving the overall quality and relevance of generative AI results by incorporating information from the organization's internal knowledge bases, document repositories and other sources.
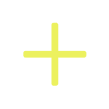
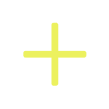
FourthLock pipelines are easy to install on the hardware of your choosing. Simply provision resources on servers that you manage on-premise or through the cloud provider of your choice. We provide the deployment deployments assets needed to depoy and manage your pipeline in a scalable Kubernetes cluster.
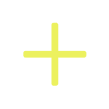
Our unified permission gateway tracks data lineage for all data sources and ensures that stringent security and governance controls are enforced at all pipeline stages. All output data formats include lineage tagging for enforcement of access controls. It's also important to note that since your entire FourthLock pipeline is deployed on your hardware, your data is never exposed to external services unless you choose to enable external access. FourthLock pipelines can be deployed can managed on fully air-gapped architectures.
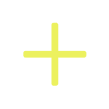
FourthLock pipelines are structured to handle multiple inputs of batch and streaming data an can output to multiple targets to support AI applications, analytics other operational needs. Most organizations start with one pipeline for multiple purposes within a given network topology. Additional pipelines can always be added based on the needs of each operation as customers scale their usage.